Dr. Otto Kolbinger
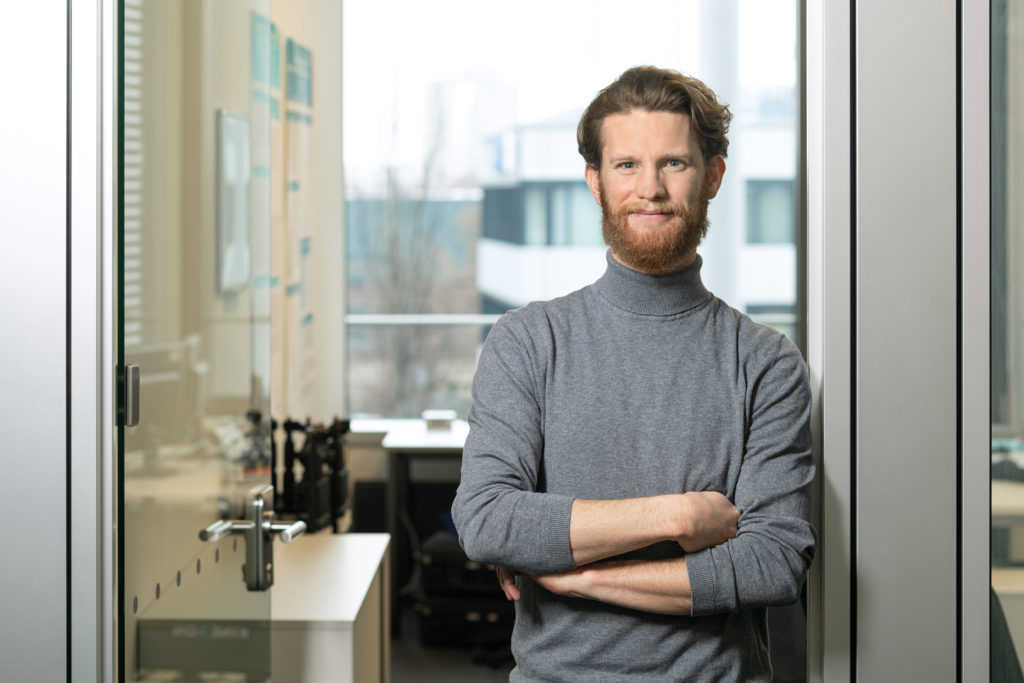
Dr. Otto Kolbinger currently works as a post-doctoral research associate at the Chair of Performance Analysis and Sport Informatics at the Technical University of Munich. His current projects focus on the evolution of rules in game sports with emphasis on trivial offenses and technological officiating aids. Further, he serves as consultant for several data science projects of a leading German sports magazine.
Invited Session: Text Mining
In sport science – and performance analysis in particular – there are various scenarios in which valuable information is provided as unstructured text data: Professional scouts provide extensive scouting reports for promising athletes, experts provide elaborated previews for upcoming events, and huge crowds share their opinions and feelings about on-going events. Deriving information from such unstructured text corpora in an effective manner is possible by deploying different text mining techniques, which already builds a significant part of the methodological spectrum in various research areas (e.g. economics, public health). In this invited session, we want to highlight how different text mining approaches have already been applied in performance analysis to detect and predict different events as well as to evaluate technological officiating aids. In addition, we give an outlook how else this innovative methodology could be deployed to provide support for practitioners in sport.
Dr. Albrecht Zimmermann
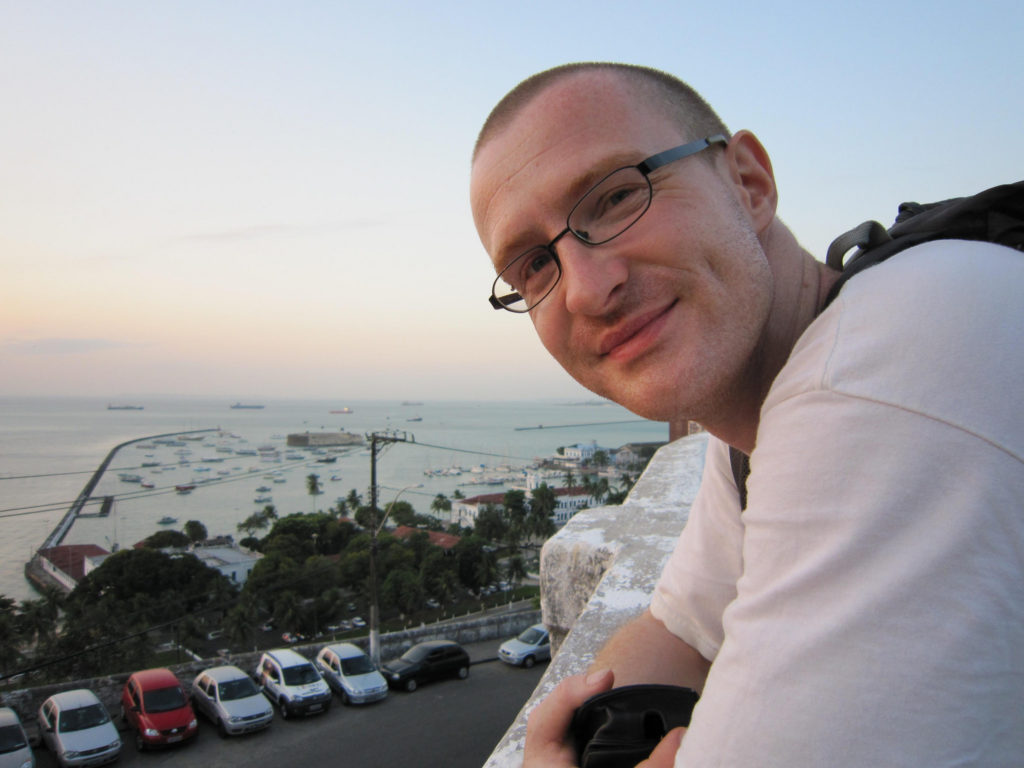
Albrecht Zimmermann ist mâitre de conférences (associate professor) at the Greyc laboratory of the University of Caen. He is co-organizer of the on-going workshop series on Machine Learning and Data Mining for Sports Analytics (MLSA). His main work is in pattern mining but his research interests span a wide range of machine learning and data mining topics.
Invited Session: Using machine learning to assess and compare athletes in team sports
Obviously, a valuable player in team sports is someone who helps their team win. At a fine-grained level, this means that such players perform actions that improve their team’s chance of winning and avoid doing those that decrease these chances. But what are good and bad actions is far from obvious, even for experts and aficionados of the sport, and looking at all actions a player is performing, and grading them, quickly becomes an insurmountable task. Machine learning allows to create models that allow deciding actions’ values, turning subjective and sporadic assessments into data driven ones. This allows assessing players in turn, whether they are valuable, particularly good (or bad) at something, and how similar they are to other players. In this session, we will discuss how different machine learning techniques have been employed to address those tasks. The resulting information can be used for game-planning, to decide which players to acquire or replace, or for selective training.
Professor Nic James and Dr Nimai Parmar
London Sport Institute, Middlesex University, London, UK. Chris Connelly, GB Boxing, English Institute of Sport, UK.
Nic is a Professor of Sport and Exercise Science and Head of Research in the London Sport Institute at Middlesex University. He has worked with England squash, GB Olympic and Paralympic teams, International dancers, professional rugby and football teams as well as coaches and athletes from other sports in multiple European countries. His research interests include notational analysis, statistics and motor learning, all of which inform his teaching on the MSc Performance Analysis. Nic has supervised 11 PhDs to completion and currently supervises 6, two of whom work for Liverpool FC and two Leicester City FC. Dr Nimai Parmar is a Senior Lecturer in Performance Analysis and Programme Leader for the MSc in Sport Performance Analysis at Middlesex University in London. Nimai has led on projects and funded studentships with multiple professional and elite sports in the UK and currently supervises 7 PhD students in performance analysis and sports science. Chris has previously provided PA support to GB Handball, Port Vale FC, Stoke City FC, The Premier League and UK Goalball. Chris now works as senior performance analyst for GB boxing as well as a Technical Lead for the English Institute of Sport. During his time with GB Boxing he has provided PA support at multiple World and European Championships, Commonwealth Games and the Rio Olympics. Chris is now leading PA provision in preparation for Tokyo Olympics. In addition to these roles, Chris heads up performance analysis support to Heavyweight World Champion boxer, Anthony Joshua.
Talk Synopsis:
This talk will cover the challenges associated with speaking the same language between computer scientists, performance analysts and coaches. In particular, identifying achievable and interesting research questions. Exemplars of projects within Squash and Boxing will be provided and the talk will discuss how these language barriers have been overcome resulting in successful outcomes for the projects and explore the lessons learned.
Prof. Dr. Daniel Memmert

Daniel Memmert is a Professor and Executive Head of the Institute of Exercise Training and Sport Informatics at the German Sport University Cologne. From 2009 to 2016, he was Institute Director at the Institute for Cognition and Sports Game Research at the German Sport University Cologne. In 2003, he received his PhD (award: dvs Young Scientist Award, bronze) and habilitated in 2008 at the Elite University of Heidelberg (award: DOSB Science Award, bronze). In 2014, he was a visiting professor at the University of Vienna. His scientific work focuses on movement science (cognition and motor function), sports psychology (attention and motivation), and computer science in sports (Big Data, pattern recognition and simulation).
According to a publicly accessible database of the world’s top 100,000 scientists (https://data.mendeley.com/datasets/btchxktzyw/2), he ranks first in Germany in the field of “Sport Science”. He has an H-index of 51 (i10-index 150) and has raised more than 8 million Euros in third-party funding (e.g. BMBF, BISp), including 6 DFG projects in the field of computer science and 5 DFG projects in the field of psychology. In addition, he has completed several research stays (e.g., USA, Canada), won various awards (e.g., DOSB Science Award Bronze, Research Writing Award AAHPERD), serves on international editorial boards, and has published more than 200 articles in international journals, 20 books, and 30 book chapters. From 2009 to 2013 he was executive director of asp (Association for Sport Psychology), from 2012 to 2016 he was editor of the Journal of Sport Science (behavioral science section), from 2016 to 2018 he was associate editor (psychology) for the journal Research Quarterly for Exercise and Sport, from 2017 to 2021 for the Journal of Sport Psychology, and since 2009 he is deputy speaker of the dvs commission “Team Sports”. He holds coaching licenses in the sports of soccer, tennis, snowboarding as well as alpine skiing and is editor and author of textbooks on modern soccer training. His institute cooperates with various Bundesliga soccer teams, the German national soccer team and DAX companies and organizes the first international master’s degree course in “Match Analysis”.
Invited Session: Interdisciplinary collaboration
Match Analysis 4.0 with Big Data: From Studies to Experiments
The lecture provides an overview of the development of match analysis in recent years. Based on technological developments in sensor technology, especially in the field of commercial football, coupled with changes in media preparation of sports games, new types of performance evaluation have been established. The massive increase in available data consolidated under the term ‘big data’ makes it possible to calculate more complex performance indicators. Based on the positional data of the individual players and the ball, analyses can be significantly faster than with video-based material. Whereas in the past the focus was on the analysis of frequencies of certain game events, it is now not only possible to calculate specific metrics, but they are applied already (Memmert & Rein, 2018). These metrics make it possible to picture the performance of teams and individual players as well as the interaction dynamics between teams (Memmert et al., 2017; Low et al., 2019). It is shown that the actual significance for the performance of many of these new performance indicators (KPIs) is often still insufficiently scientifically proven (Memmert & Raabe, 2018). In one of the largest big data field studies conducted so far, the DFL-funded big data field study (Memmert et al., 2016) therefore defined various KPIs in professional football and validated them in the first steps. The lecture offers an outlook on why it is necessary to develop models in order to specify the link between big data and a “match analysis 4.0” (Rein & Memmert, 2016) and to make the resulting hypotheses empirically verifiable through field experiments based on positional data. Such an experimental paradigm would be appealing, as it would be able to generate real data in an 11 vs. 11 football game, theory-guided (not post-hoc testing), reliable, objective with corresponding KPIs, and extremely fast.